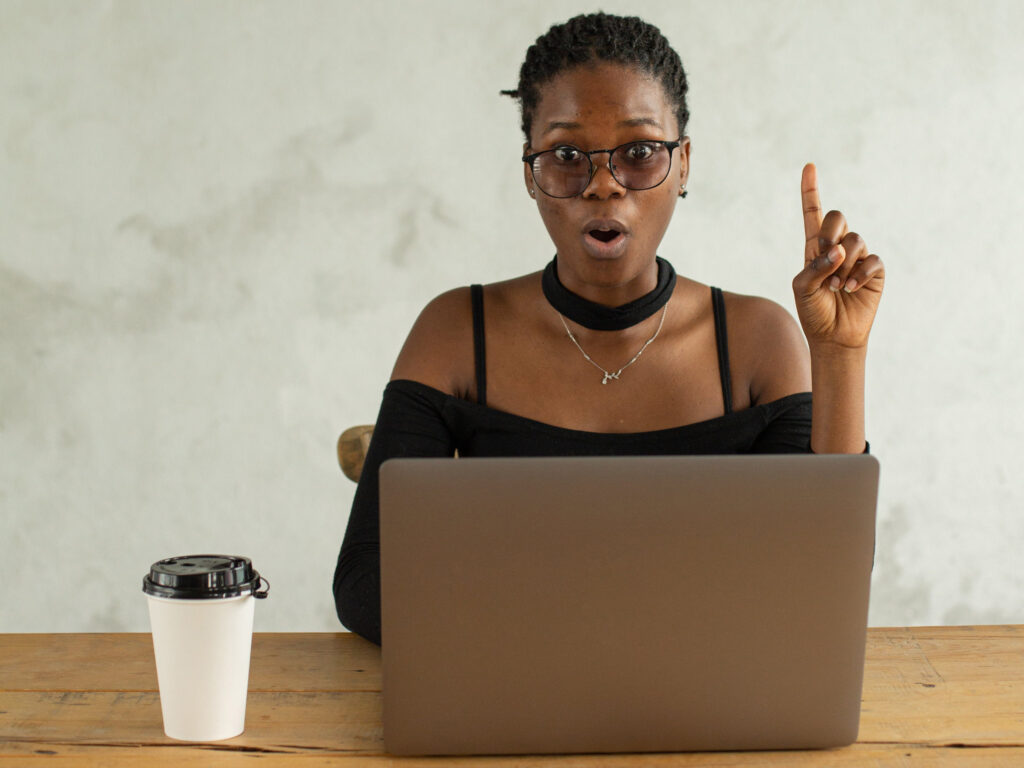
The third value of the Agile Marketing Manifesto is “Learning through experiments and data over opinions and conventions.” Experiments and data are better than opinions and doing things like we’ve always done (conventions). But sometimes, data isn’t enough – we also need insights.
Without insights, we don’t make giant leaps forward. We don’t create new markets that didn’t exist before. And we’re in danger from new competitors who have insights, even if data don’t back up their vision. In short, there is a cost to being data-driven vs insight-driven.
Thin Data vs Thick Data
Social scientists distinguish between “thin data” and “thick data.” Thin data is quantitative data about populations. Thin data is sometimes referred to as statistics or Big Data. Thick data provides context. It is qualitative. For good decisions, marketers need both thin data and thick data.
For example, Netflix is known for its recommendation engine. Most of the recommendation engine is based on thin data, quantitative data about what you’ve previously watched. But Netflix also invests in thick data. It hired a well-known cultural anthropologist named Grant McCracken as well as investing in an internal consumer preferences team.
They gather information that thin data can’t provide: what are people doing while watching a show, sitting on the couch or exercising? How do people interact with each other while watching? How do they react to recommendations?
When they watched people’s viewing habits in their living rooms, one of the behaviors they identified was binge-viewing, watching episode after episode of a series in a short period. When they returned to their data models, they could see the binge-watching, but until they observed it, they did not glean this insight from their data. The combination of thick data, backed up by thin data, led to Netflix’s decision to release the episodes of a new series all at once rather than over time.
Steve Blank, a serial entrepreneur who became a professor of entrepreneurship at Stanford University and the author of “The Startup Owner’s Manual,” is famous for his dictum: Rule No. 1: There Are No Facts Inside Your Building, So Get Outside. Steve required his entrepreneurship students to talk to at least 100 customers about their ideas for a business before they built anything. Essentially, Steve advocated gathering thick data to provide insights before committing to an idea.
Data Analysis vs Data Synthesis
Data analysis is the process of breaking down data and looking at the pieces individually. Data synthesis combines data from different quantitative and qualitative sources, thick and thin, in new and creative ways to derive meaning.
Both data analysis and data synthesis are essential, but data synthesis is the more critical skill for marketers, who are often faced with uncertainty and asked to anticipate the future rather than report on the past.
Imagine being a marketer in 2010 at Blackberry, the Canadian company behind the Blackberry mobile device. You show the following sales data:
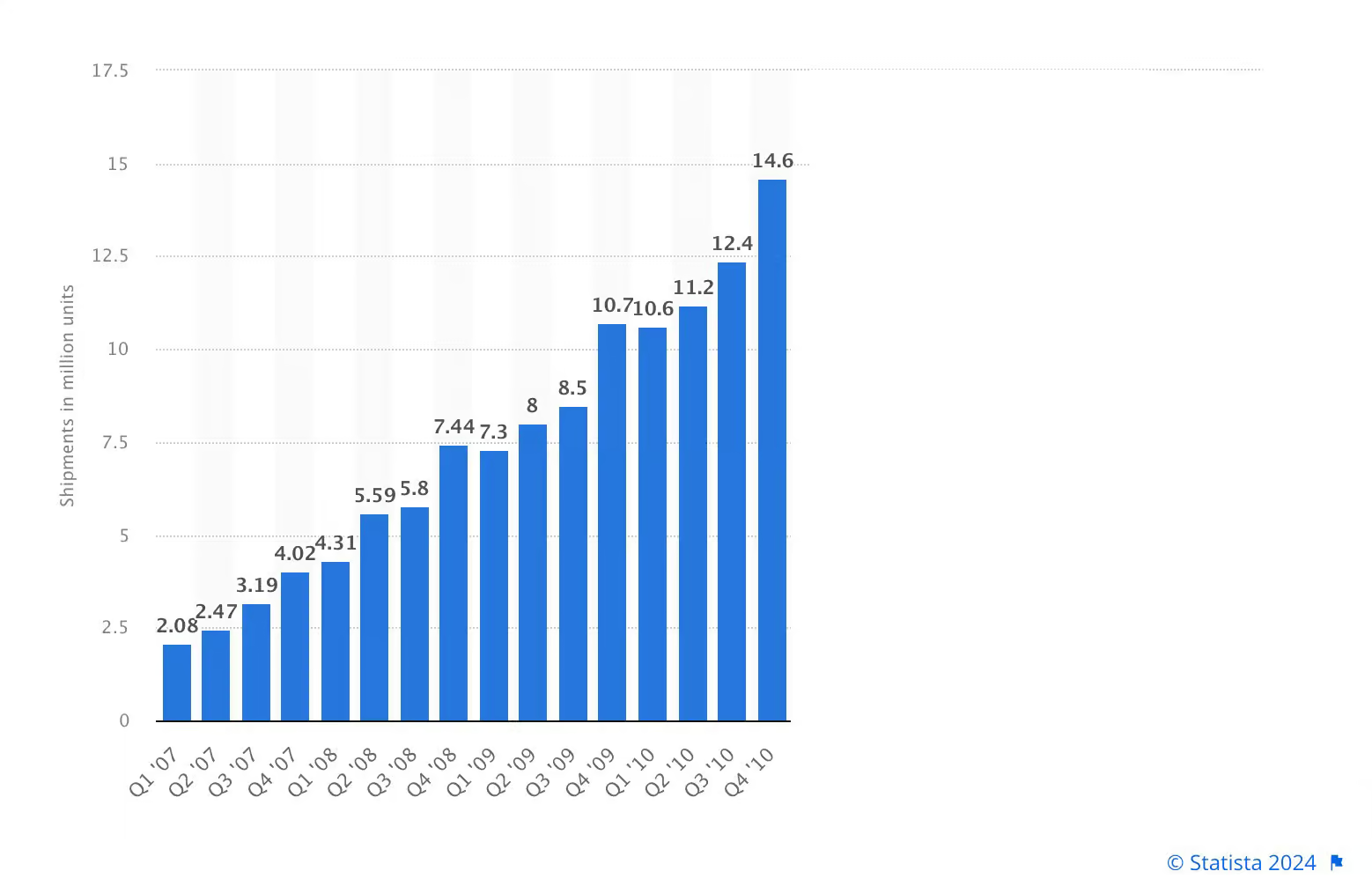
Although Apple launched the first iPhone in Q2 of 2007, Blackberry sales continue to climb. You’ve just had a fantastic Q4.
But what if you did some data synthesis and told a story with a different data set, such as the number of applications available for iPhones vs. Blackberries? In 2010, Apple had over 300,000 apps in the App Store, compared to just 18,000 for Blackberry. Furthermore, imagine you showed the executive team interviews with iPhone users about how much they loved specific applications.
You might have avoided this:
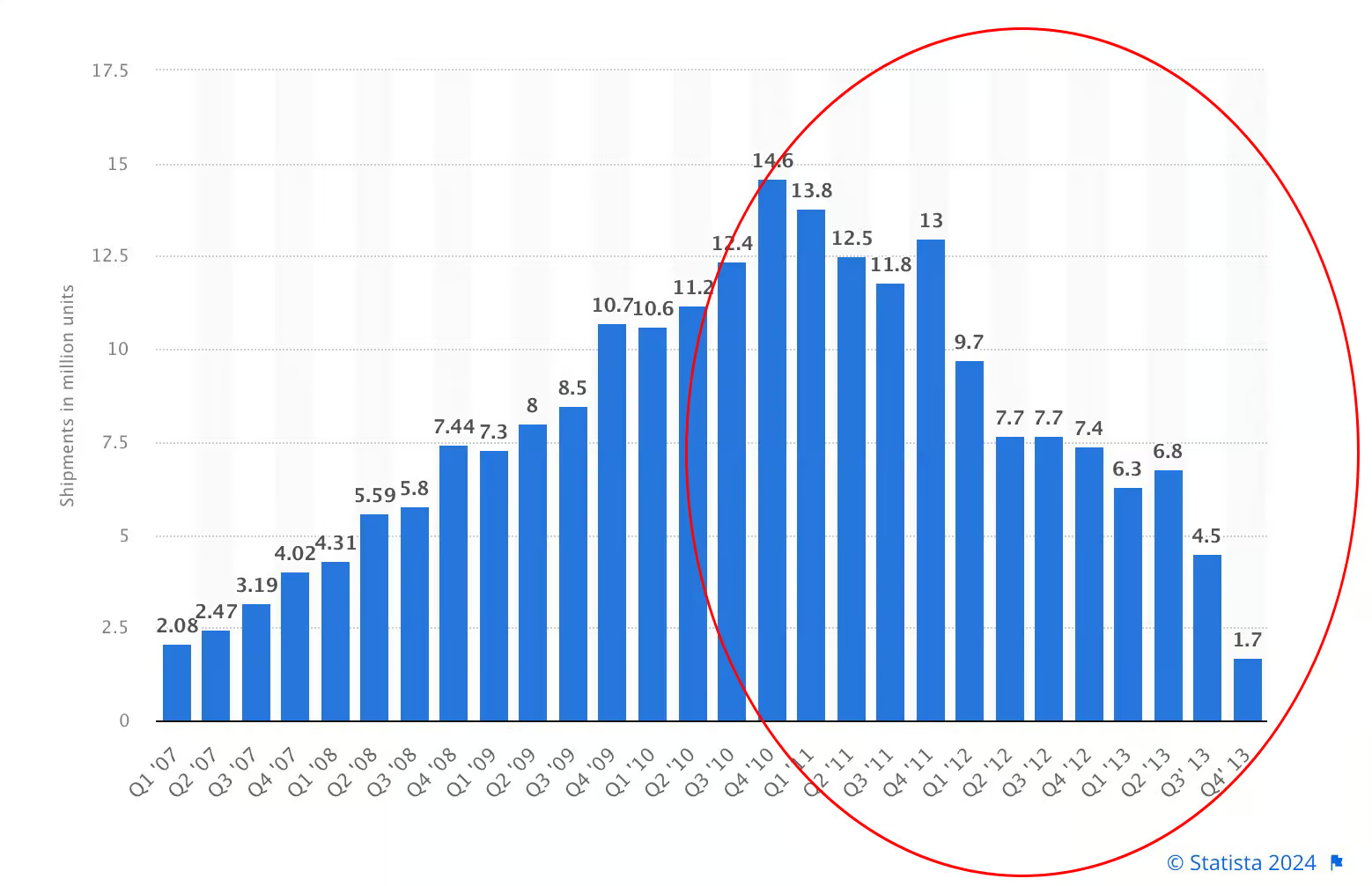
Being Insight-Driven Over Data-Driven
Being insight-driven rather than data-driven represents a fundamental shift in how organizations approach decision-making and strategy. Here’s what it entails:
Focus on Understanding Over Mere Data Collection
While data-driven organizations prioritize collecting and analyzing large volumes of data, insight-driven organizations focus more on understanding and interpreting this data to glean meaningful insights. It’s not just about having data; it’s about understanding what the data is telling you.
Qualitative Combined with Quantitative Data
Data-driven approaches often lean heavily on quantitative data — numbers, metrics, and statistics. Insight-driven approaches, on the other hand, place significant value on qualitative data — observations, experiences, and subjective interpretations. This involves looking at the reasons behind trends, the stories data tells, and the human elements that numbers alone can’t capture. You need both.
Creativity and Innovation
Being insight-driven encourages more creativity and innovation. Insights often come from thinking outside the box, challenging assumptions, and connecting dots in novel ways. It involves interpreting data regarding market trends, consumer behavior, and other external factors.
Human Judgment and Expertise
While data-driven models can heavily rely on algorithms and machine learning, insight-driven approaches emphasize human judgment and expertise. It recognizes that data interpretation often requires a human touch — understanding nuance, context, and subtleties that machines may miss.
Holistic View of Data
An insight-driven approach takes a more holistic view of data. It integrates and synthesizes data from various sources, including market trends, customer feedback, and internal metrics, to form a comprehensive understanding.
Conclusion
There’s a cost to just being data-driven in making marketing decisions. Making good marketing decisions involves combining qualitative and quantitative data, thick and thin data. It also involves the human touch: creativity, innovation, and insight. By being insight-driven, marketers will better understand where customers are going rather than just where they’ve been.
As Wayne Gretzky, one of the greatest hockey players of all time, famously said “I skate to where the puck is going to be, not to where it has been.”