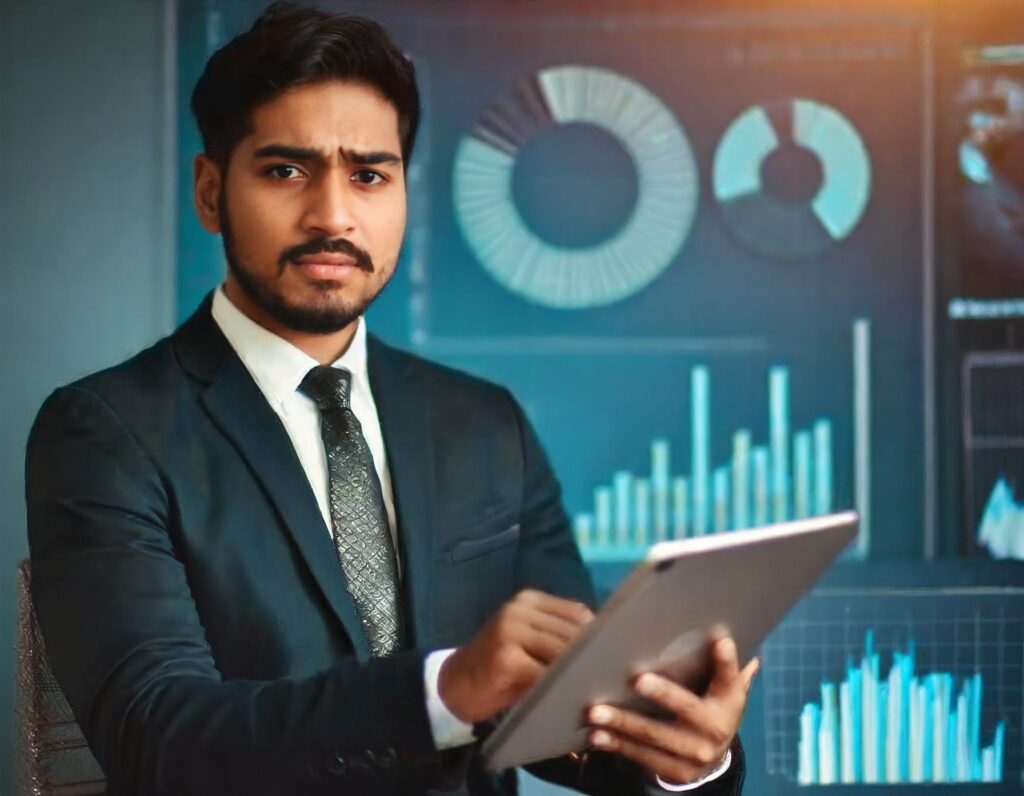
Marketers want to understand how to improve their campaigns’ conversions and ROI. They often want to test multiple factors and multiple choices for each factor. For example, a marketer might want to test 3 different prices, 3 different headline messages, and 2 different design approaches. To test all of those variables, they would have to run 18 different tests, as shown in the table below:
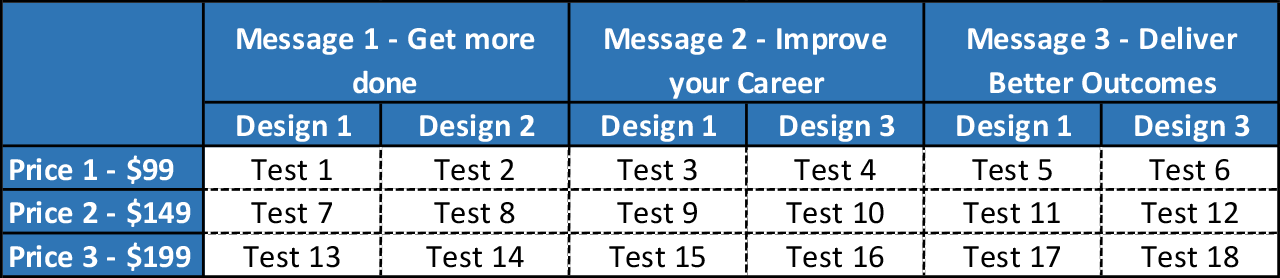
While this can be done, it is complex to run 18 different tests. In order to achieve some kind of statistical significance, it might also need a lot of traffic. For example, if each cell requires a sample size of at least 10,000 visitors, then to test all of these variations would take a minimum of 180,000 visitors. Unless you have very high web traffic, achieving statistical significance might take longer than you’re willing to wait to get an answer.
This approach to testing all the different permutations of multiple variables is called full factorial multivariate testing. That’s a mouthful! This approach is sometimes shortened to full factorial MVT.
The challenge with full factorial multivariate testing is that it requires a lot of work and a lot of traffic to test. So for most marketers, who may not have unlimited amounts of traffic, they need to select the right experimental design for their situation.
Selecting the Right Experimental Design for a Low-Traffic Website
While full factorial MVT is the gold standard, there are alternatives for sites with less traffic and needing answers in a reasonable time frame.
Focus First on the Highest-Impact Changes
Depending on your audience, you may decide that either design or pricing are the factors that are most likely to have the highest impact on your conversion rate. If you choose pricing, you might decide to run an A/B test, or in this case, an A/B/C test.
A/B Testing
A/B testing, also known as split testing, is the simplest form of testing in digital marketing. It involves comparing two versions of a webpage or app against each other to determine which one performs better. You change one variable (in this case price) while keeping other elements constant.
The advantage of A/B testing is its simplicity in implementation and analysis. It is ideal for small-scale changes like testing two different prices or two different colors of a conversion button. The primary disadvantage is that it is limited to testing one variable and just 2 or 3 variations of that one variable.
Split URL Testing
Split URL testing is similar to A/B testing but is used when the changes are significant enough to warrant separate URLs. For example, testing two entirely different landing page designs. Everything is kept the same (price and messaging) except the design.
Split URL testing is suitable for testing major redesigns or functional changes and provides more flexibility in design and structure. The major disadvantage is that it requires more traffic to achieve statistical significance due to separate URLs.
Try Fractional Factorial Multivariate Testing
Fractional (sometimes called partial) Factorial MVT testing tests only a portion of all possible combinations of Full Factorial MVT Testing. For example, in our example above, Fractional MVT might run only 9 of the tests above, inferring the results of the other 9 tests through some complex statistical analysis.
While technically not as comprehensive as Full Factorial MVT testing, it is usually good enough for marketers with lower-traffic websites or digital campaigns.
Use Fewer Variations
While testing as many variations as possible is tempting, marketers with lower-traffic websites may need to use fewer variations. In our example, the marketers could choose to test just two price points and two messages, reducing the number of tests to 8 rather than 18.
Consider Lowering Your Level for Statistical Significance
Scientists typically require a minimum of 95 percent or sometimes 99 percent statistical significance. Marketers may want to consider not waiting for the test to reach these levels of statistical significance. If your testing tool allows, lower your statistical significance levels to 80% or even 70%. Any version that reaches this mark will become the winner. In this case, you would also require a much smaller sample size than going for 99% significance.
Conducting Market Experiments Testing
Here are some suggested steps for conducting marketing testing:
1. Identify a Focus Area
Select a specific aspect of your marketing to test and optimize it before moving on to other areas. This could be a webpage, an email campaign, or an app interface. This decision should be guided by your marketing objectives and areas where you suspect improvements can be made.
2. Define Goals and Hypotheses
Set clear, measurable goals for your testing (e.g., increase conversion rates by X%). Formulate hypotheses based on your understanding of your audience and previous marketing data. For instance, hypothesize how changing the color and text of a call-to-action button might affect user behavior.
3. Create Variations
Develop different versions of your focus area. In web design, for instance, you might alter elements like headlines, images, button colors, and layout. Ensure that variations are distinct enough to glean meaningful data.
4. Determine Sample Size
Use statistical tools to calculate the sample size necessary for reliable results. This depends on your current traffic, conversion rates, and the number of variations you plan to test.
5. Run Tests
Employ tools like VWO, AB Tasty, or Optimizely to implement your test. Ensure the test runs for a sufficient duration to gather actionable data, typically until statistical significance is achieved.
6. Drive Traffic
Ensure consistent traffic to your test variations for accurate results. Use your standard marketing channels like SEO, paid ads, or social media, to drive traffic, ensuring that the sample is representative of your typical audience.
7. Analyze Results
Analyze the collected data to understand which combination of variables worked best. Look at metrics like click-through rate (CTR), conversion rates, engagement, and time on page.
8. Iterate
Based on the results, implement the most successful combinations and consider retesting with new variations. This iterative process is crucial for continuous improvement and optimization.
When to Use Which Testing Method
- A/B Testing: Best for testing minor changes where you want quick, clear results.
- Split URL Testing: Ideal for major redesigns where changes are too extensive for a single page.
- Full Factorial Multivariate Testing: Use when you want to understand how multiple elements interact and are willing to invest more time and resources for comprehensive insights.
- Fractional Factorial Multivariate Testing: when you want the advantages of full factorial multivariate testing but you only have the traffic and the resources to do a partial or fractional test.
Tools for Testing: VWO, AB Tasty, and Optimizely
VWO (Visual Website Optimizer)
Advantages:
- User-friendly interface
- Has a free version for getting started and light traffic websites
- Offers a range of testing options including A/B, full factorial multivariate testing, fractional factorial multivariate testing, and split URL.
- Offers heatmap analytics
Disadvantages:
- The paid versions get very expensive quickly
- Some users report a steep learning curve.
AB Tasty
Advantages:
- Great for both beginners and advanced users.
- Offers personalization features alongside testing.
Disadvantages:
- Limited integrations compared to other platforms.
- Reporting can be overwhelming for new users.
Optimizely
Advantages:
- Robust testing capabilities.
- Strong analytics and reporting features.
Disadvantages:
- Higher cost, making it less accessible for smaller companies.
- Complex setup that might require technical expertise.
Conclusion
It’s not easy to test multiple factors and multiple choices for most marketers. To make the best of the resources they have and the traffic that they can drive, it’s important to choose the right experimental design. Whether you use A/B testing, Split URL testing, full factorial multivariate testing, or fractioinal factorial multivariate testing, choose the design that is best for your particular marketing circumstances.